SimCorp Data Warehouse: is it worth it?
- Feb 27, 2024
- 8 min read
In the era of big data, businesses are inundated with information from various sources. The challenge lies not just in collecting this data, but in storing, managing, and making sense of it. This is where the concept of a Data Warehouse comes into play.
A Data Warehouse serves as more than just a storage system — it's a critical element of business intelligence, playing a key role in strategic decision-making by embodying the business data model. It acts as a central repository where data from multiple sources is consolidated, transformed, and stored. This data can then be retrieved, analyzed, and turned into actionable insights, of course dependent on the quality and completeness of this business data model.
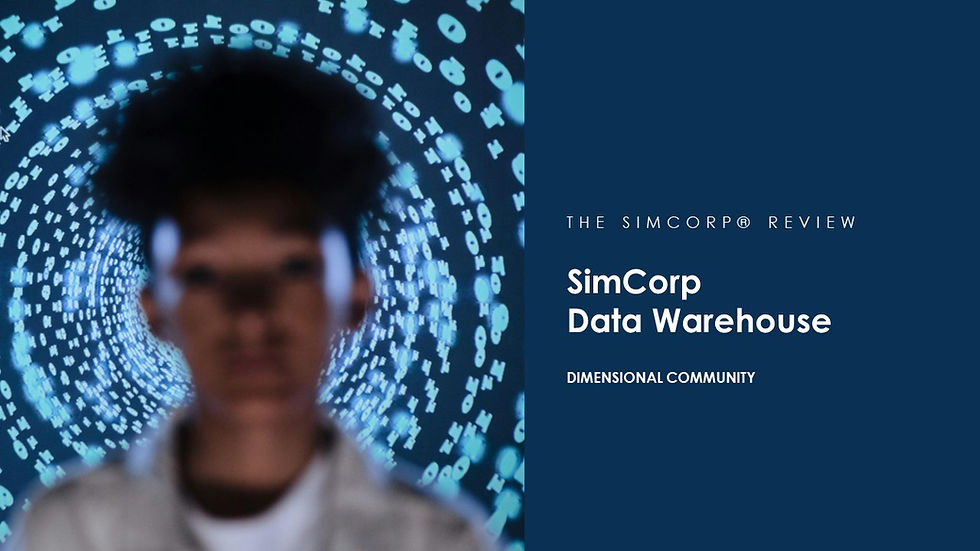
This blog delves into SimCorp's Data Warehouse solution, tracing its origins and examining the strategic partnership with Snowflake. The maturity of this collaboration is evaluated, highlighting key features such as the mesh architecture facilitated by data sharing and the marketplace. Additionally, the discussion encompasses decision intelligence, conversational analytics, and the implementation of automated analytics, providing a comprehensive overview of SimCorp's evolving data strategy.
The beginning: The IMW
The data model in SimCorp Dimension can be challenging to work with because the model is highly normalized. While this secures non-redundant and standardized data, the result is often complex queries to extract data. Faced with this challenge, SimCorp realized back in 2014 that they needed to address this issue and introduced the SimCorp Datawarehouse (DWH).
A solution within the Oracle Database was created, which offers a flattened view based on a methodology developed by Ralph Kimball. This approach ensures that the facts within the Dimension view of the data are highly structured and easily consumable. This structured and simplified view provides a more accessible and user-friendly experience, enabling users to derive meaningful business insights and abstractions from the data.
SimCorp refers to this solution as the Investment Management Warehouse – or IMW for short.
The architectural structure of data in the IMW model is centered around domain data, particularly focusing on the investment flow. This means that the data is organized in a way that aligns with key stages of the investment process, including investment planning, investment execution monitoring, and investment administration. This architectural approach is designed to provide a coherent and logical framework for managing and analyzing data throughout the various phases of the investment management lifecycle.
Common data sets such as the Investment Book of Record (IBOR) serve as a foundational element supporting various investment processes within the system. Additionally, there are shared data sets encompassing information like securities, party classifications, ratings, and similar elements. These shared datasets ensure consistency and coherence across different domains, promoting efficient data management and analysis.
While the IMW is fully integrated with SimCorp Dimension, it allows for augmentation of data through the inclusion of 3rd-party data sources — a practice widely adopted by SimCorp clients.
New Horizons: Snowflake
In 2022, as SimCorp strategically embraced a "cloud-first" approach for their technology stack, they formed a partnership with Snowflake as their selected technology provider for cloud-native capabilities. This decision was driven by several factors: the platform's cloud-agnostic framework, a consumption-based pricing model, and a notable highlight — the ability to leverage the data marketplace.
Snowflake's architecture is designed to be approached as a data mesh, where the emphasis is on making all data accessible without necessitating central consolidation. By leaving the data at its source and adopting a mesh architecture, end consumers naturally gain greater accessibility to the data, leading to increased value and consumption. Moreover, with more data readily available, users are more inclined to leverage it, resulting in increased consumption.
Some major benefits of Snowflake when compared to traditional storage, is not only a swifter delivery of data to the end consumer but also a reduction in the total cost of ownership, including reduction in data costs – some reports up to 30 times thanks to its efficient data footprint.
While SimCorp Dimension remains the core investment platform, the IMW deployed with Snowflake can be considered as a seamless complement, ensuring data accessibility for diverse consumption needs. This ranges from direct querying, allowing data scientists to use Python for analysis, to more managed analytics through Business Intelligence (BI) tools. Here It should be noted that SimCorp also offers client reporting through a digital engagement platform portal, which facilitates reporting for SimCorp’s clients' clients, aligned with the cloud-native warehouse.
For clients where SimCorp is hosting in the cloud under SaaS and clients themselves adopt a Snowflake strategy, they can leverage the benefits of data sharing which allows secure sharing of data across different business ecosystems. Or put differently: Snowflake-to-Snowflake interoperability. SimCorp oversees the extraction of data from Dimension into Snowflake, managing the pipeline, changes, and the evolution of the data model. This approach empowers clients to specify how they want to derive business value from the data through data sharing. Outcomes have been significant, with notable benefits in terms of SLAs, accelerated data access, heightened resilience, and, of course, a reduction in total cost of ownership.
It is clear from speaking with SimCorp and attending their conferences that the integration with Snowflake is significant in shaping SimCorp’s future roadmap and that they equally see it as crucial for their clients to refine and advance their data strategies.
Maturity of offering
More than 100 SimCorp clients are using the DWH solution. SimCorp’s new partnership with Snowflake has shown good interest, and a few clients are in production. We expect that the uptick in clients interested in Snowflake to grow in the coming years.
Whether clients are using the Oracle data warehouse structure or SimCorp's cloud solution, they can opt to migrate to the Snowflake Warehouse with minimal effort, contingent on the level of customizations undertaken in the past. If the client adhered to the standard model, the migration is expected to be relatively straightforward.
Mesh Architecture: Through Data Share & The Marketplace
It is a strategic focus of SimCorp to develop more cloud-native components, and one of the recent additions is Analytics as a Service which we discussed in November 2022 in this SimCorp Review where it was called Performance Analytics as a Service. This is a cloud-native offering that is meant to eventually replace the existing on-premises version of SimCorp’s Performance Manager.
This simplifies processes and reduces the need to generate extensive data on various aggregation levels for performance reports and store it within SimCorp. Instead, the Analytics Service functions as a self-service cloud-native solution, storing results in memory and fully enabled with API’s. Only performance numbers that need to be reported on are stored in the database.
The most recent development in Analytics as a Service is the integration piece. This entails a comprehensive workflow that involves accessing APIs, transferring data down to Snowflake through the cloud warehouse within a defined structure, and subsequently enabling Data Share. That means data is not only confined to the main SimCorp Dimension on-premise installation, i.e., the Oracle footprint. Instead, cloud-native services, both current and future offerings, can also be accessed within Snowflake.
Through Data Share, SimCorp clients can enhance their organizational data within their ecosystem while leveraging the Snowflake Marketplace to integrate third-party data seamlessly. With live access, not a copy of the data. This approach aligns with a mesh architecture, allowing all end users to access the data within a unified data plane.
Decision Intelligence – Access to the Data Plane
SimCorp is departing from the traditional model of prescribed dashboards where someone creates a dashboard and end users filter data. Taking inspiration from generative AI (Artificial Intelligence) trends, an integrated BI solution now features a cloud-native capability. The solution is hosted by SimCorp, offering accessibility from anywhere — be it on mobile devices, tablets, in the office or at home. Features are immediately available upon development, eliminating the need for a maintenance window concept.
This shift in technology means that business users can achieve key outcomes without the need to engage IT. For instance, proactive alerting is a feature that allows analytics users to set up alerts for specific metrics, by configuring these alerts within the intelligence tool, specifying tolerances and recipients. Data can be scheduled, and collaboration extended to third parties, seeking their interest and opinions on particular metrics in a two-way collaboration. Additionally, the system seamlessly integrates with machine models and AI within its engine.
Taking a closer look at AI, the BI tool includes an inside advisor grounded in two core principles. The first principle revolves around an associative engine, an approach where data is not filtered upon entry to this layer. Instead, all data remains accessible for analytics, embracing an associative rather than filtered methodology. This means no data is excluded, whether it's joined or un-joined, providing a comprehensive foundation for analytics.
Complementing this, the second principle incorporates the power of AI to support a diverse range of use cases. From expediting the creation of charts to facilitating advanced analysis, the AI integration is designed to enhance user experience. This includes leveraging Natural Language Processing (NLP) for conversational analytics, enabling users to visualize data based on their questions. Furthermore, the AI capabilities delve into uncovering hidden relationships within the data and even extend to suggesting and forecasting analytics. Together, these principles create a dynamic and intelligent BI tool.
Conversational Analytics
Conversational analytics allows users to engage with data in an entirely new way - by asking natural language questions in plain simple text, bypassing the need for predetermined dashboards. For instance, one might inquire about a portfolio’s performance since inception. The engine, powered by machine learning and AI, translates these inquiries into visual representations (e.g. graphs, diagrams). Further, users can refine their queries, such as breaking down the information by sector.
The beauty of this system lies in its accessibility; no developers or IT are required. The process seamlessly integrates conversation analytics, allowing users to delve into data without technical barriers. This conversational analytics model extends its capabilities to leverage data from various sources within Snowflake, exemplifying its adaptability and efficiency.
Automated Analytics
Another advantage of generative AI SimCorp is taking advantage of is automated analytics. This involves the system scrutinizing the data and proactively proposing insights. These generated insights stem directly from the provided data, allowing users to filter and specify their focus. For instance, one might zoom in on a particular data set for Europe, prompting the system to furnish generated automated analytics in a conversational, natural language response.
Pushing the boundaries even further, users can invoke generative AI to enhance their selections. A user might instruct the system to leverage generative AI by specifying parameters, such as CO2 emissions for a particular GICS sector. This prompts the system to reach out to external sources, such as OpenAI, for insights beyond the presented data. This integration expands the analytical horizon, incorporating not only the user's data but also insights from external AI capabilities.
Bottom Line
SimCorp's Data Warehouse solution has matured considerably over the past decade, transforming into a robust tool that facilitates more straightforward and efficient reporting. The recent integration with Snowflake adds a layer of sophistication, aligning seamlessly with SimCorp's broader cloud and services strategy. This flexibility caters to a diverse range of client preferences, whether they opt for an on-premise or a cloud-based solution.
The introduction of conversational analytics represents a significant leap in user-friendliness, allowing stakeholders to interact with data effortlessly. Furthermore, automated analytics, driven by generative AI, takes a proactive approach by suggesting insights based on the provided data. This not only streamlines analytical processes but also expands the horizon of possibilities for users, fostering a more sophisticated and insightful data-driven environment.
We trust that this blog post has provided you with valuable insights. Whether you're exploring the solution, currently using it and eager to share your experiences, or if you have any questions, we welcome your engagement and would appreciate hearing from you.